Monitor available IPs with Lambda and Cloudwatch
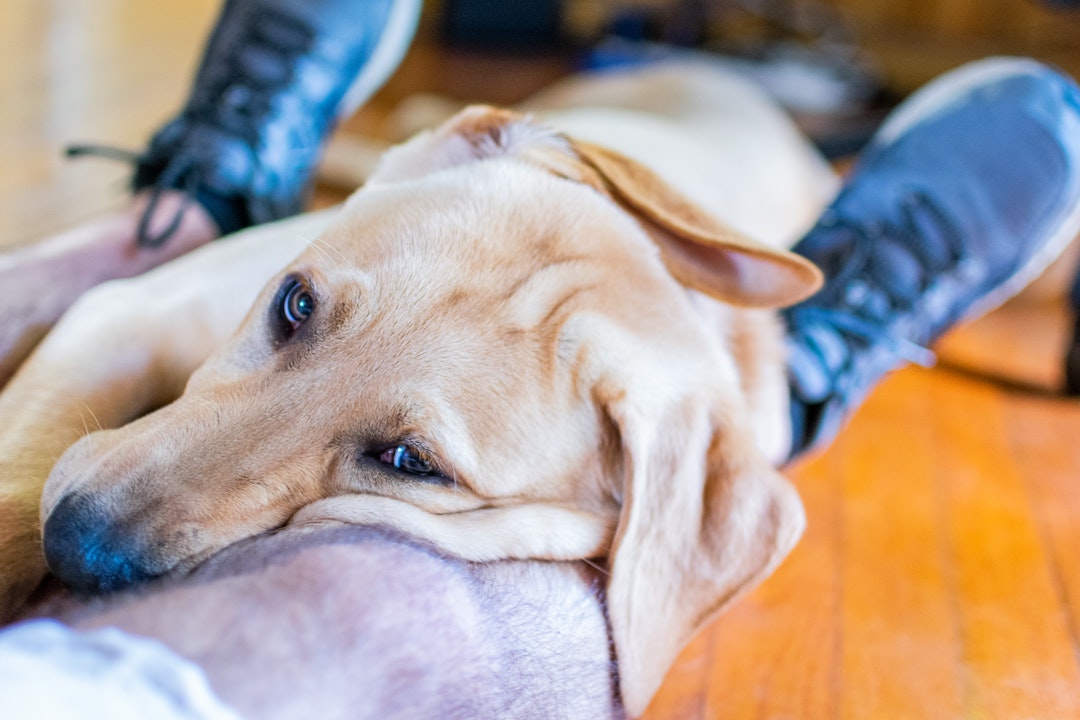
I ran into a situation where I needed to keep track of available IPs related to an AWS EKS cluster and couldn’t find any off the shelf tooling in AWS or otherwise to do so.
Tangential gripe: The reason I needed the monitor is because EKS doesn’t support adding subnets to a cluster without re-creating it and the initial subnets that were used were a little too small due to reasons.
Do NOT use wildcard alternate domains in AWS CloudFront
CloudFront configs allow for alternate domain names if you’d like to use a custom domain for your CDN distribution. You likely want that.
The alternate domain list can include wildcard subdomains, like *.example.com – see the docs. This is handy for dev and experiment environments so you don’t have to be constantly updating the config.
Imagine you have a scenario where you have a Route53 (DNS) & CloudFront config that looks like:
Frequently asked tech career questions
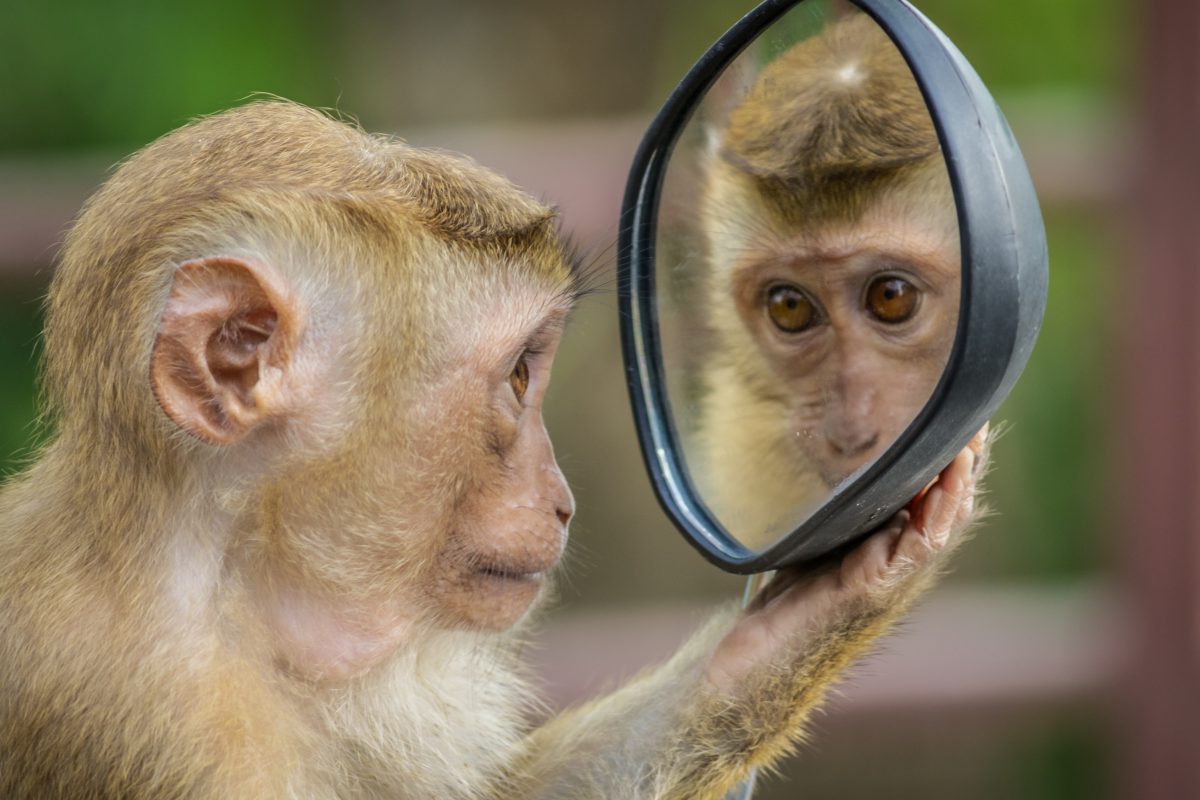
I’ve reached the point in my career and have enough gray hair that people sometimes ask me for career advice. Usually they are in tech, trying to make a transition into a different role, or are trying to get into tech. I do my best to answer these questions because it is obvious that they have experienced some sort of trauma and/or have run out of better options if they are at the point where they can look into the melancholy and barely obscured madness behind my eyes and think “This is someone who can help me.
Which Kubernetes Container Probe Should I Use?
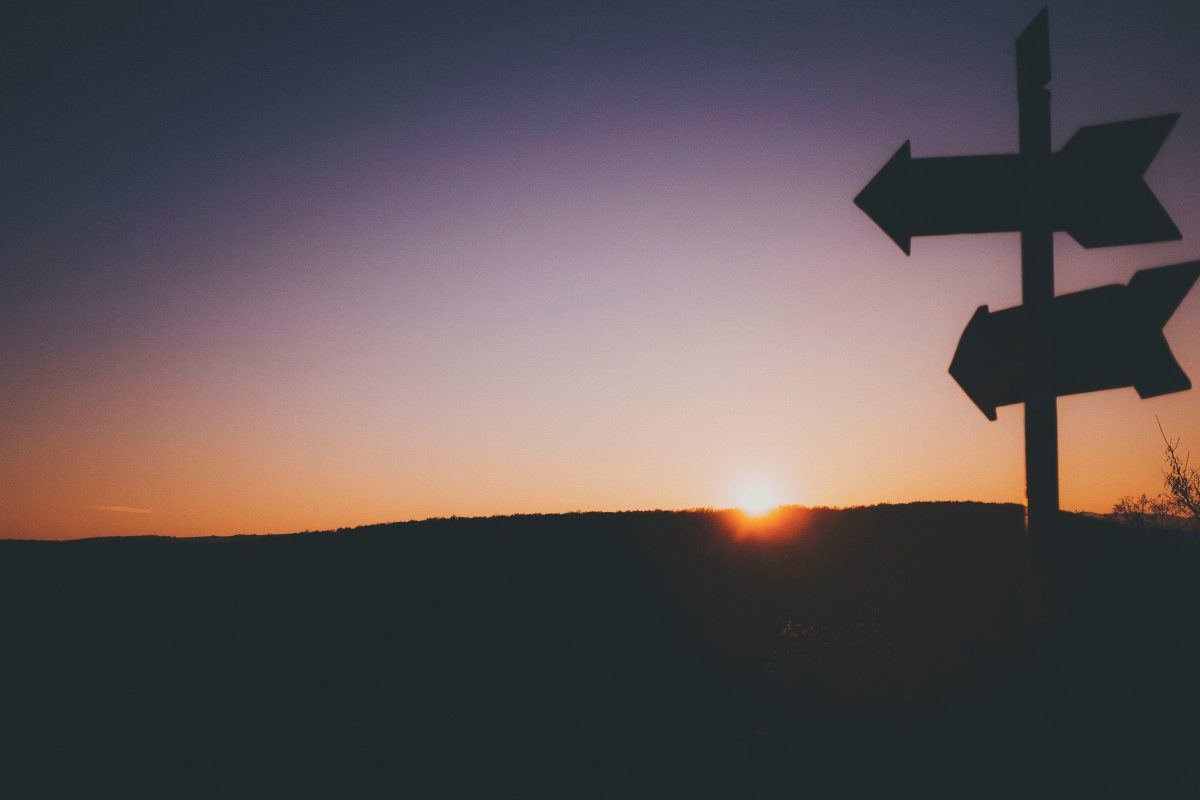
As you lean into your Kubernetes (k8s) cluster’s self-healing capabilities, one of the first concepts you’ll encounter is that of readiness, liveness, and startup probes. These can be a little confusing because there is a bit of overlap between them. There’s also a lot of risk in getting your configuration wrong, so it’s an area you’ll want to put significant thought towards.
What are probes in this context? k8s probes are effectively abstractions of health checks.
You are not your code
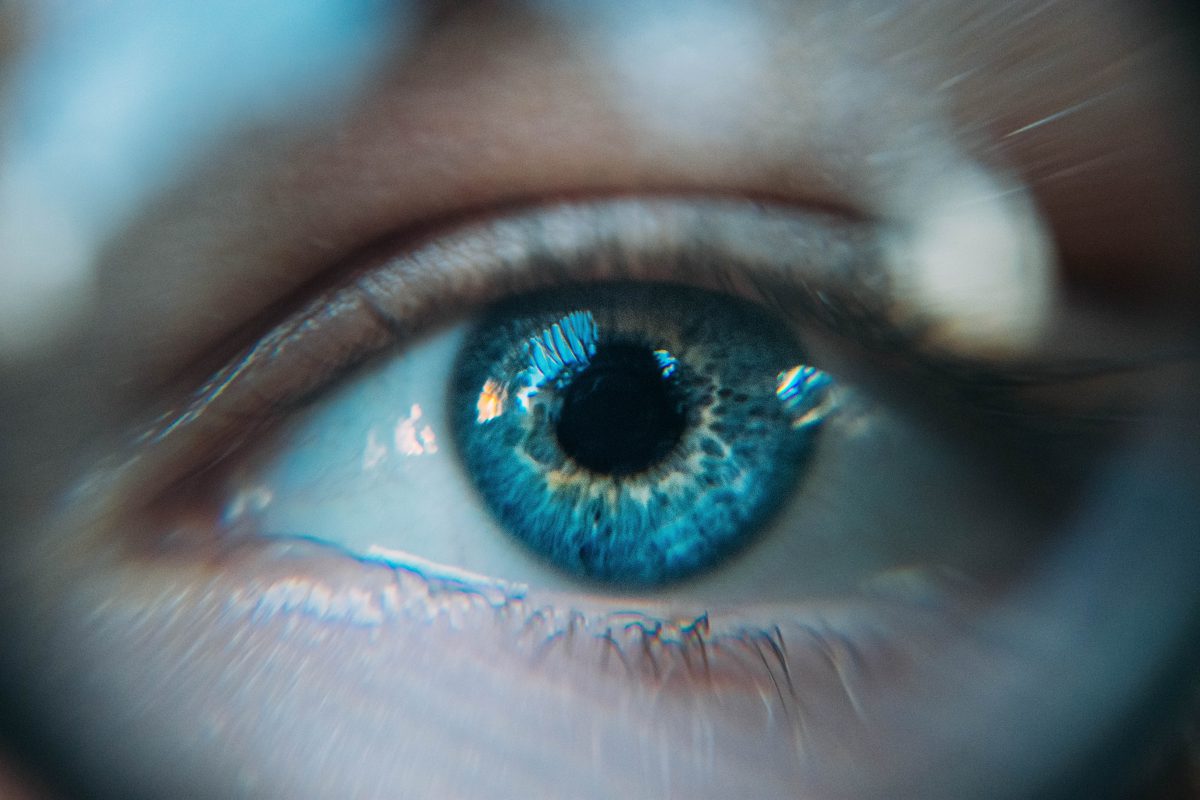
You… are not your code. You are not your configs, your documentation, your blog posts, your conference talks, or your job.
You. Are. Not. Your. Work.
I say this as someone who spent many years of my life deriving personal value from the work I produced. It started in angst-y teenage writing – “No one understands me. These words are my true self.” – one of the dumbest of youthful ideas, possibly only surpassed by “I need to hurt to create.
You probably shouldn’t be using Kubernetes for your new startup
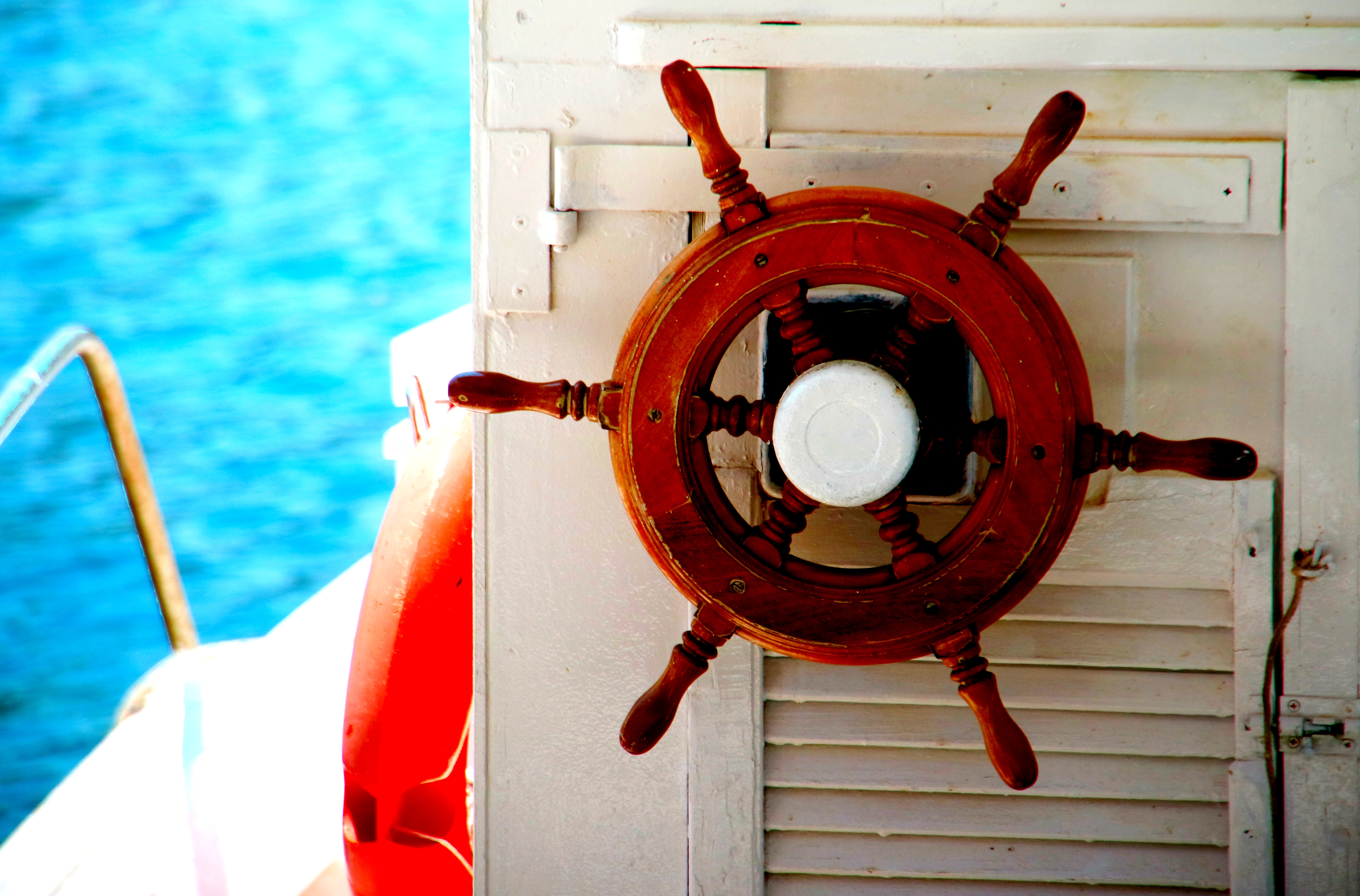
Kubernetes (k8s) is awesome. I am a fan. I like it so much I have a k8s-themed license plate on my car, but I cringe every time I see a blog post or tweet pitching it as a solution for a nascent company.
Like microservices (Which, strong opinion incoming… you also probably shouldn’t be using, especially for something new. Seriously, stop. ), k8s solves a specific set of problems (mostly coordination/abstraction of some infrastructure components/ deployments and a lot of stuff related to general scaling/self-healing) and comes with significant, usually overlooked cost.
TIL: How to live-rotate PostgreSQL credentials
OK, I didn’t actually learn this today, but it wasn’t that long ago.
Postgres creds rotation is straightforward with the exception of the PG maintainers deciding in recent years that words don’t mean anything while designing their identity model. “Users” and “Groups” used to exist in PG, but were replaced in version 8.1 with the “Role” construct.
Here’s a map to translate PG identifies to a model that will make sense for anyone who is familiar with literally any other identity system.
Kubernetes EC2 autoscaling for fun and profit
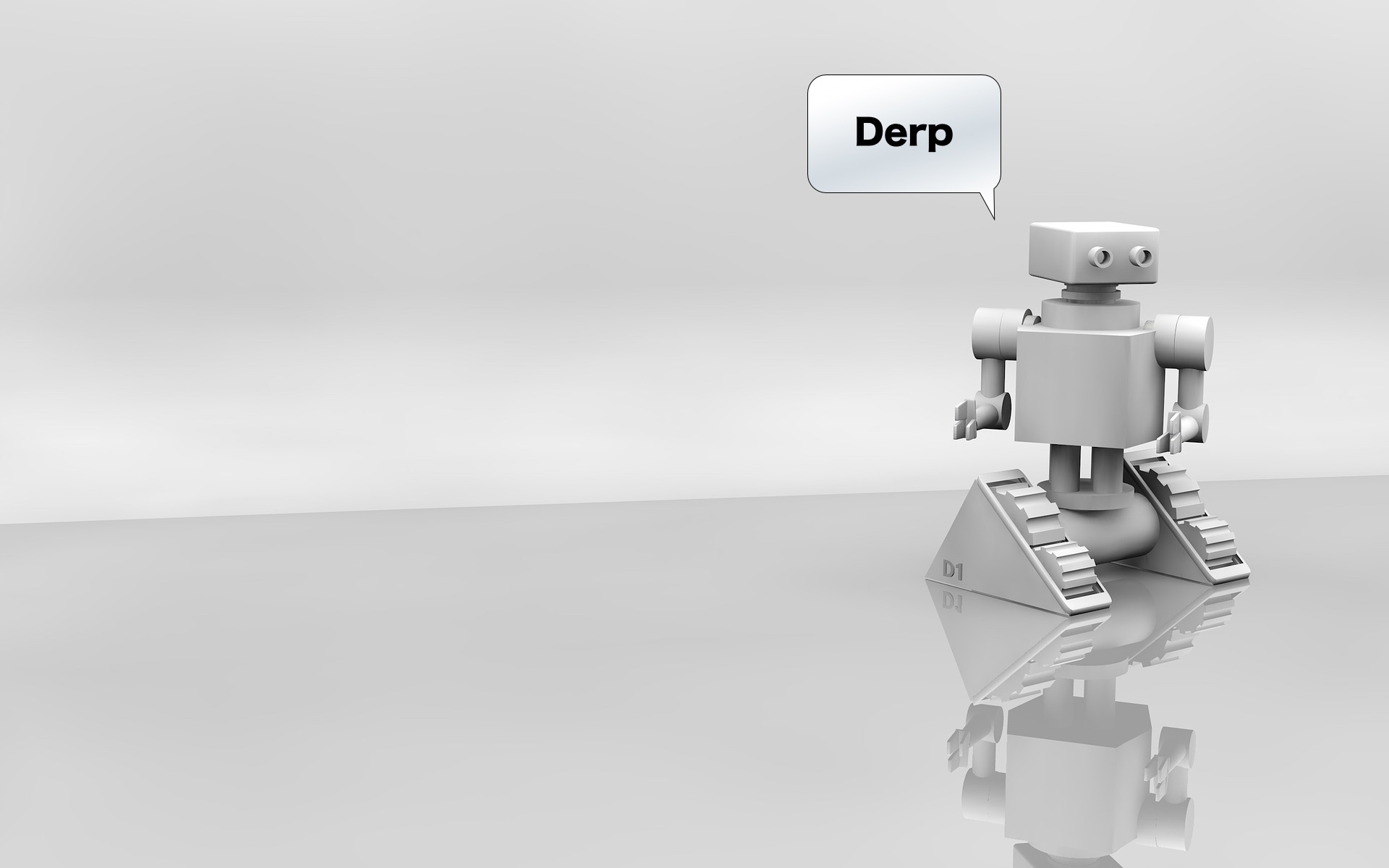
I’m drawn to the puzzles of distributed systems and abstracted platforms – the problems that only crop up when lots of moving pieces work in tandem (or not!).
I recently encountered one of these issues a few weeks after a platform migration to AWS EKS.
The symptoms The initial problem manifested itself as an application async worker issue.
Async process queues began stacking up and triggering alerts. Investigation of the worker process revealed that: Workers reported healthy Workers seemed to be processing the maximum number of threads per worker Workers were using minimal compute resources Some of the queue was getting processed Re-deploying the async worker Kubernetes (k8s) pods resolved the immediate problem and the queues started draining again.
What I love about SRE
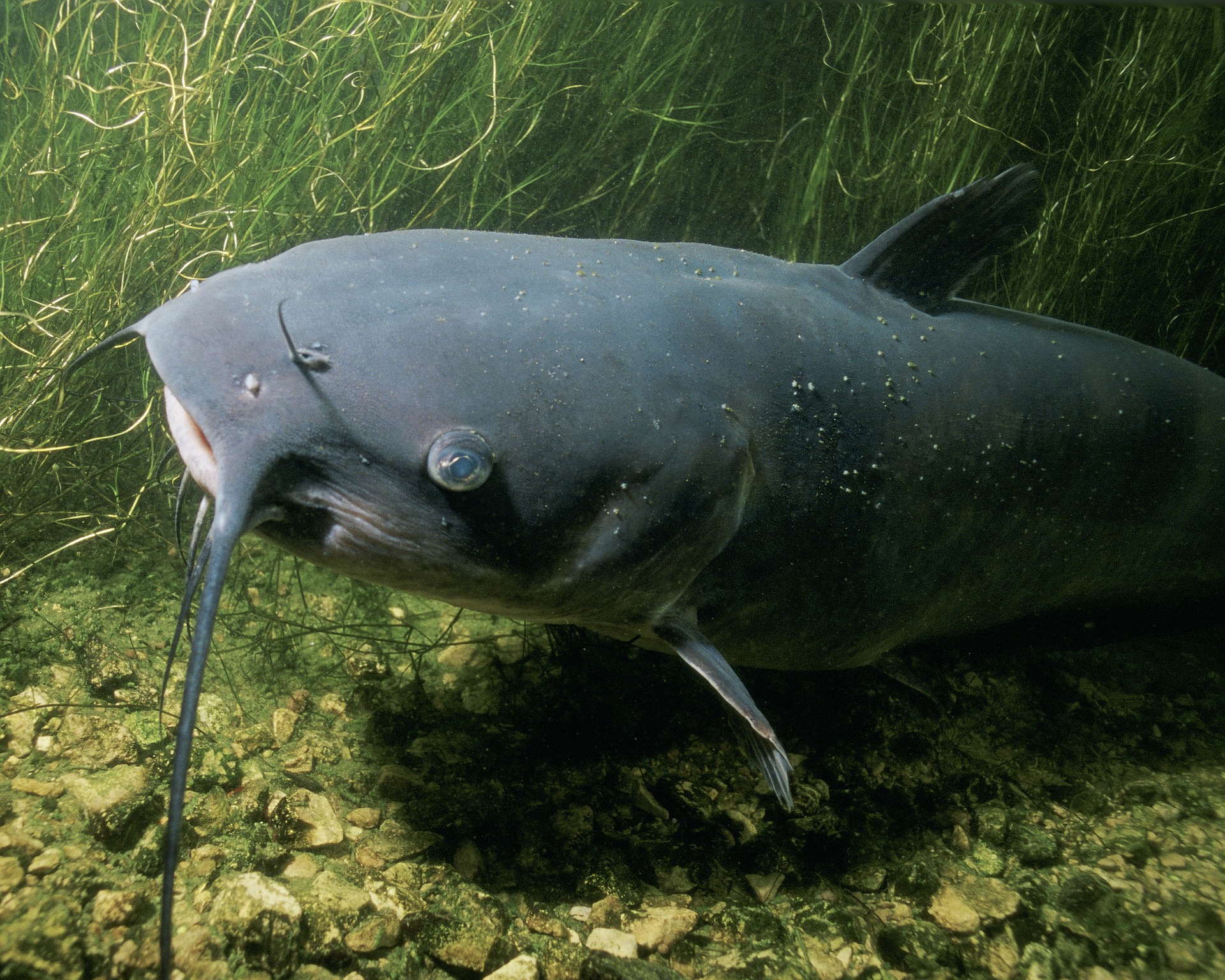
My childhood was soaked in science. As I learned the alphabet and how to tie my shoes, my dad spent his days taking water samples and caring for the fish that made up the research cohort for the aquaculture study he ran. We lived at a research site on the lake and I toddled along through three hot summers, staring into the eyes of whiskered catfish and witnessing the hard, mundane work of science interwoven through our daily lives.
Books from Q4 2018
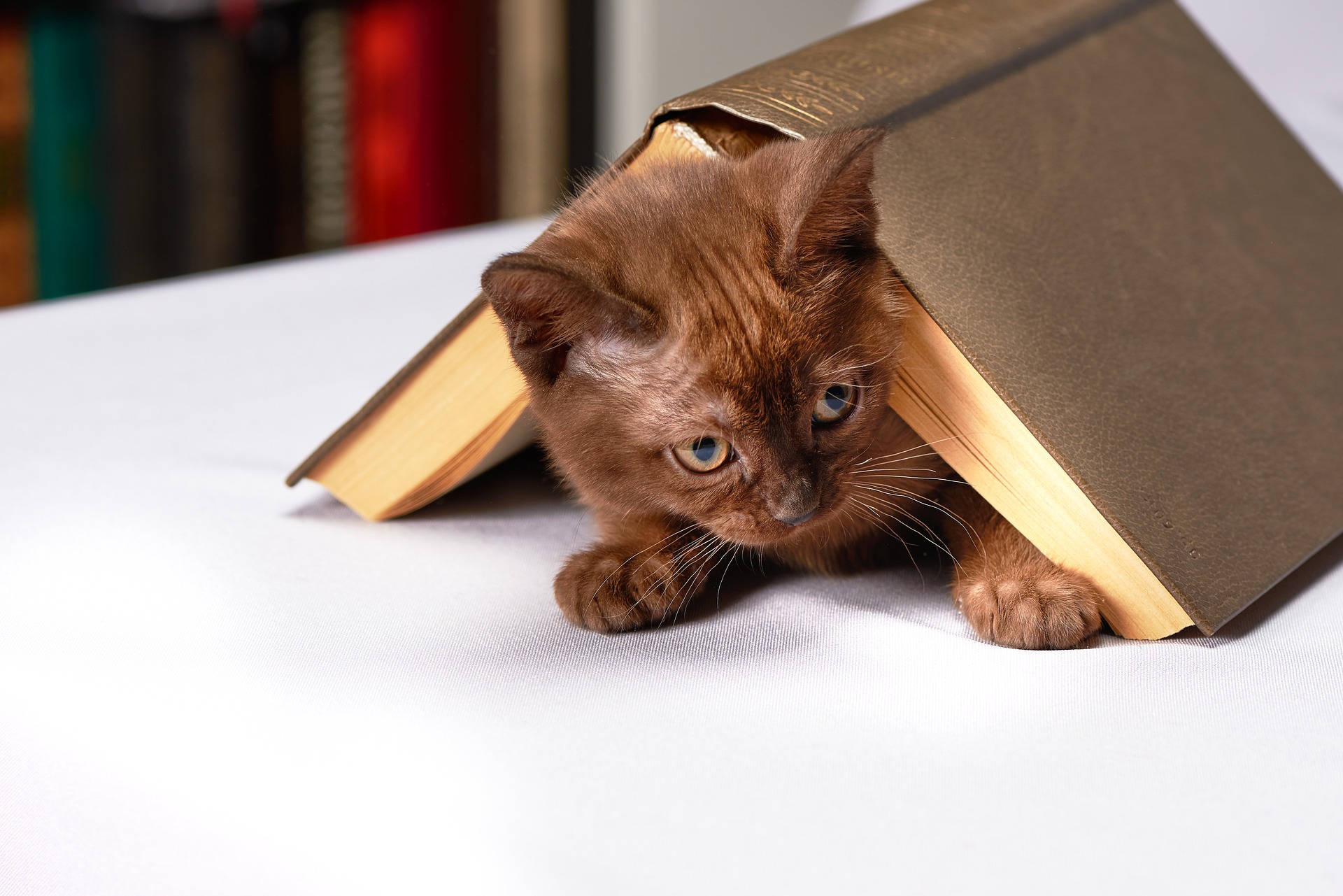
Continuing from Q1, Q2, & Q3.
48. “The Worst Journey in the World” – Apsley Cherry-Garrard
The title of this book is pretty accurate. It covers the Terra Nova Expedition to the South Pole during 1910-1913 – a failed race (Roald Amundsen won) that resulted not only in reaching the pole late, but in the death of the main expedition teams. Overall, it’s a very un-even book and could have used a more forceful editor.